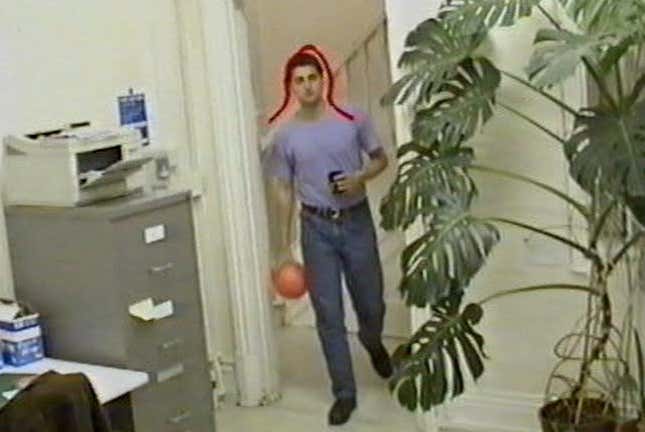
In 1998, I accidentally created a racially biased synthetic intelligence set of rules. There are classes in that tale that resonate much more strongly these days.
The risks of bias and errors in AI algorithms are actually widely recognized. Why, then, has there been a flurry of blunders through tech firms in contemporary months, particularly on this planet of AI chatbots and symbol turbines? Preliminary variations of ChatGPT produced racist output. The DALL-E 2 and Strong Diffusion symbol turbines each confirmed racial bias within the photos they created.
My very own epiphany as a white male computer scientist happened whilst educating a pc science magnificence in 2021. The category had simply seen a video poem through Pleasure Buolamwini, AI researcher and artist and the self-described poet of code. Her 2019 video poem “AI, Ain’t I a Woman?” is a devastating three-minute exposé of racial and gender biases in automated face reputation techniques – techniques evolved through tech firms like Google and Microsoft.
The techniques ceaselessly fail on girls of colour, incorrectly labeling them as male. One of the crucial disasters are in particular egregious: The hair of Black civil rights chief Ida B. Wells is categorized as a “coonskin cap”; some other Black girl is categorized as possessing a “walrus mustache.”
Echoing over the years
I had a terrible déjà vu second in that laptop science magnificence: I all at once remembered that I, too, had as soon as created a racially biased set of rules. In 1998, I used to be a doctoral scholar. My challenge concerned monitoring the actions of an individual’s head in accordance with enter from a video digital camera. My doctoral adviser had already evolved mathematical techniques for correctly following the top in positive scenarios, however the device had to be a lot sooner and extra tough. Previous within the Nineteen Nineties, researchers in other labs had proven that skin-colored spaces of a picture might be extracted in genuine time. So we made up our minds to concentrate on pores and skin colour as an extra cue for the tracker.
I used a virtual digital camera – nonetheless a rarity at the moment – to take a couple of photographs of my very own hand and face, and I additionally snapped the palms and faces of 2 or 3 different individuals who came about to be within the development. It was once simple to manually extract one of the skin-colored pixels from those photographs and assemble a statistical fashion for the surface colours. After some tweaking and debugging, we had an incredibly tough real-time head-tracking system.
No longer lengthy later on, my adviser requested me to reveal the device to a couple visiting corporate executives. After they walked into the room, I used to be in an instant flooded with nervousness: the executives had been Jap. In my informal experiment to peer if a easy statistical fashion would paintings with our prototype, I had amassed knowledge from myself and a handful of others who came about to be within the development. However 100% of those topics had “white” pores and skin; the Jap executives didn’t.
Miraculously, the device labored fairly effectively at the executives anyway. However I used to be surprised through the conclusion that I had created a racially biased device that can have simply failed for different nonwhite folks.
Privilege and priorities
How and why do well-educated, well-intentioned scientists produce biased AI techniques? Sociological theories of privilege supply one helpful lens.
Ten years ahead of I created the head-tracking device, the student Peggy McIntosh proposed the theory of an “invisible knapsack” carried round through white folks. Within the knapsack is a treasure trove of privileges corresponding to “I will do effectively in a difficult scenario with out being known as a credit score to my race,” and “I will criticize our executive and speak about how a lot I worry its insurance policies and behaviour with out being observed as a cultural outsider.”
Within the age of AI, that knapsack wishes some new pieces, corresponding to “AI techniques gained’t give deficient effects on account of my race.” The invisible knapsack of a white scientist would additionally want: “I will increase an AI device primarily based by myself look, and know it’ll paintings effectively for many of my customers.”
AI researcher and artist Pleasure Buolamwini’s video poem ‘AI, Ain’t I a Lady?’
One steered treatment for white privilege is to be actively anti-racist. For the 1998 head-tracking device, it would appear glaring that the anti-racist treatment is to regard all pores and skin colours similarly. Unquestionably, we will be able to and must make sure that the device’s coaching knowledge represents the variety of all pores and skin colours as similarly as conceivable.
Sadly, this doesn’t be sure that all pores and skin colours noticed through the device can be handled similarly. The device should classify each and every conceivable colour as pores and skin or nonskin. Subsequently, there exist colours proper at the boundary between pores and skin and nonskin – a area laptop scientists name the verdict boundary. An individual whose pores and skin colour crosses over this choice boundary can be labeled incorrectly.
Scientists additionally face an uncongenial unconscious predicament when incorporating variety into mechanical device studying fashions: Various, inclusive fashions carry out worse than slim fashions.
A easy analogy can provide an explanation for this. Consider you’re given a decision between two duties. Activity A is to spot one specific form of tree – say, elm timber. Activity B is to spot 5 sorts of timber: elm, ash, locust, beech and walnut. It’s glaring that in case you are given a hard and fast period of time to follow, you are going to carry out higher on Activity A than Activity B.
In the similar method, an set of rules that tracks best white pores and skin can be extra correct than an set of rules that tracks the overall vary of human pores and skin colours. Despite the fact that they’re acutely aware of the desire for variety and equity, scientists will also be subconsciously suffering from this competing want for accuracy.
Hidden within the numbers
My introduction of a biased set of rules was once inconsiderate and probably offensive. Much more regarding, this incident demonstrates how bias can stay hid deep inside an AI device. To look why, believe a selected set of 12 numbers in a matrix of 3 rows and 4 columns. Do they appear racist? The pinnacle-tracking set of rules I evolved in 1998 is managed through a matrix like this, which describes the surface colour fashion. Nevertheless it’s unimaginable to inform from those numbers on my own that that is in truth a racist matrix. They’re simply numbers, decided mechanically through a pc program.
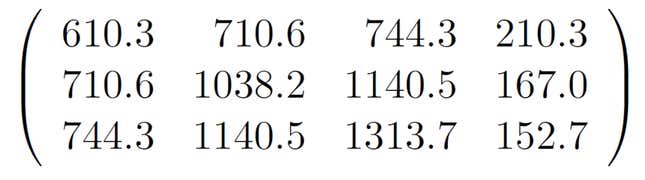
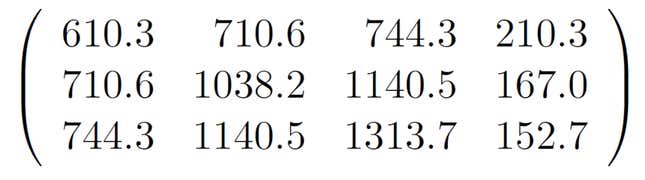
The issue of bias hiding in undeniable sight is a lot more critical in fashionable machine-learning techniques. Deep neural networks – recently the most well liked and robust form of AI fashion – ceaselessly have thousands and thousands of numbers through which bias might be encoded. The biased face reputation techniques critiqued in “AI, Ain’t I a Lady?” are all deep neural networks.
The excellent news is that a substantial amount of development on AI equity has already been made, each in academia and in business. Microsoft, for instance, has a analysis staff referred to as FATE, dedicated to Equity, Responsibility, Transparency and Ethics in AI. A number one machine-learning convention, NeurIPS, has detailed ethics guidelines, together with an eight-point listing of unfavourable social affects that should be thought to be through researchers who post papers.
Who’s within the room is who’s on the desk
Then again, even in 2023, equity can nonetheless be the sufferer of aggressive pressures in academia and business. The unsuitable Bard and Bing chatbots from Google and Microsoft are contemporary proof of this grim truth. The industrial necessity of creating marketplace percentage resulted in the untimely liberate of those techniques.
The techniques be afflicted by precisely the similar issues as my 1998 head tracker. Their coaching knowledge is biased. They’re designed through an unrepresentative staff. They face the mathematical impossibility of treating all classes similarly. They should one way or the other industry accuracy for equity. And their biases are hiding in the back of thousands and thousands of inscrutable numerical parameters.
So, how a ways has the AI box in point of fact come because it was once conceivable, over 25 years in the past, for a doctoral scholar to design and submit the result of a racially biased set of rules and not using a obvious oversight or penalties? It’s transparent that biased AI techniques can nonetheless be created accidentally and simply. It’s additionally transparent that the prejudice in those techniques will also be damaging, onerous to discover or even tougher to do away with.
In this day and age it’s a cliché to mention business and academia want numerous teams of folks “within the room” designing those algorithms. It could be useful if the sector may achieve that time. However if truth be told, with North American laptop science doctoral methods graduating best about 23% female, and 3% Black and Latino students, there’ll proceed to be many rooms and lots of algorithms through which underrepresented teams don’t seem to be represented in any respect.
That’s why the basic classes of my 1998 head tracker are much more necessary these days: It’s simple to screw up, it’s simple for bias to go into undetected, and everybody within the room is liable for fighting it.
Need to know extra about AI, chatbots, and the way forward for mechanical device studying? Take a look at our complete protection of artificial intelligence, or browse our guides to The Best Free AI Art Generators and Everything We Know About OpenAI’s ChatGPT.
John MacCormick, Professor of Laptop Science, Dickinson College
This text is republished from The Conversation below a Inventive Commons license. Learn the original article.